Project Goal
The project’s objective is to produce synthetic data mirroring specific characteristics of patients. This data will facilitate the calculation of outcome probabilities and counterfactual scenarios for different treatment options. Key anticipated outcomes include both potential side effects and prevention effects. The project places particular emphasis on patients with multimorbidity, aiming to enhance understanding and management of their complex health situations.
Background
Multimorbidity, characterized by the simultaneous occurrence of multiple chronic conditions in a single patient, poses a formidable challenge in modern healthcare, significantly impacting both treatment efficacy and healthcare costs. Traditional research methods, often reductionist in nature, inadequately address the complexities of treating such patients, as they typically focus on individual diseases. There is a critical need to adopt a more integrative approach, taking into account the interactions between various conditions and their treatments. We plan to utilize extensive observational data through advanced Machine Learning techniques, especially generative models and reinforcement learning. The same architecture we are studying is aimed at improving the data processing and analysis workflow at the LHCb experiment.
Progress
We have applied the Causal Inference framework to model the interactions between patients, treatments, and outcomes, which is essential for avoiding biases due to confounders. Our focus is on two types of data: synthetic, enabling precise mapping of relationships, and real data of varying quality. High-quality clinical trial data often have limited statistics, while observational data, though statistically rich, are noisier and less reliable.
Our strategy involves progressively incorporating noisier data. Generating epidemiologically inspired synthetic data, we’ve employed various architectures for analysis, with Conditional Variational Autoencoders being particularly noteworthy. This approach has been extended to real clinical trial data for HIV patients, assessing the benefits and risks of statin use, showing promising alignment with traditional methods.
Next, we plan to integrate reinforcement learning for causal graph modification and discovery. Additionally, we’re adapting these methods for data quality monitoring within the LHCb collaboration. Automating these tasks can significantly enhance data collection efficiency and reduce the need for manual labor. This becomes challenging during new detector commissioning, where algorithms require continuous retraining. We propose applying Reinforcement Learning with human feedback for efficient and effective anomaly detection, balancing data-collection efficiency with human factors.
Next Steps
Moving forward, our goal is to expand the causal graph’s complexity by incorporating a broader range of treatments and outcomes (both benefits and harms). We’ll assess the efficacy of generative models for different causal graphs, adjusting their structures through reinforcement learning. This evaluation will initially employ synthetic data, with prospects of applying it to real data.
Simultaneously, we’ll deepen our studies on the LHCb monitoring assistant, previously tested with synthetic data. The next step involves comparing its performance with data taken in Run-1 and Run-2.
Project Coordinator: Nicola Serra
Technical Team: Olivia Jullian Parra, Shrija Rajen Sheth, Sara Zoccheddu
Collaboration Liaisons: Professor Milo Puham (University of Zurich), Dr. Henock Yebyo (University of Zurich)
In partnership with: Roche
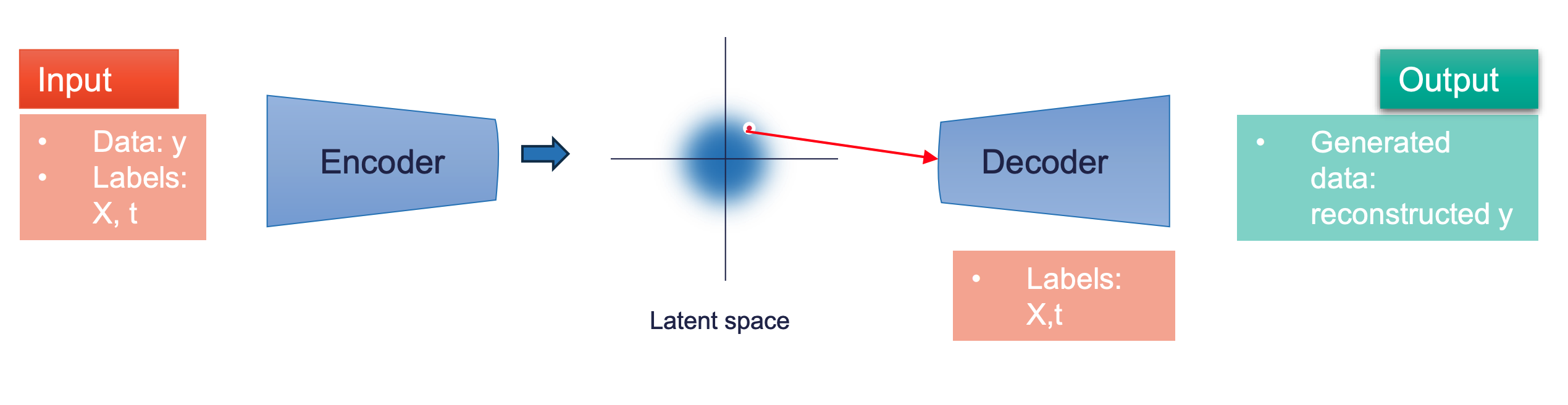